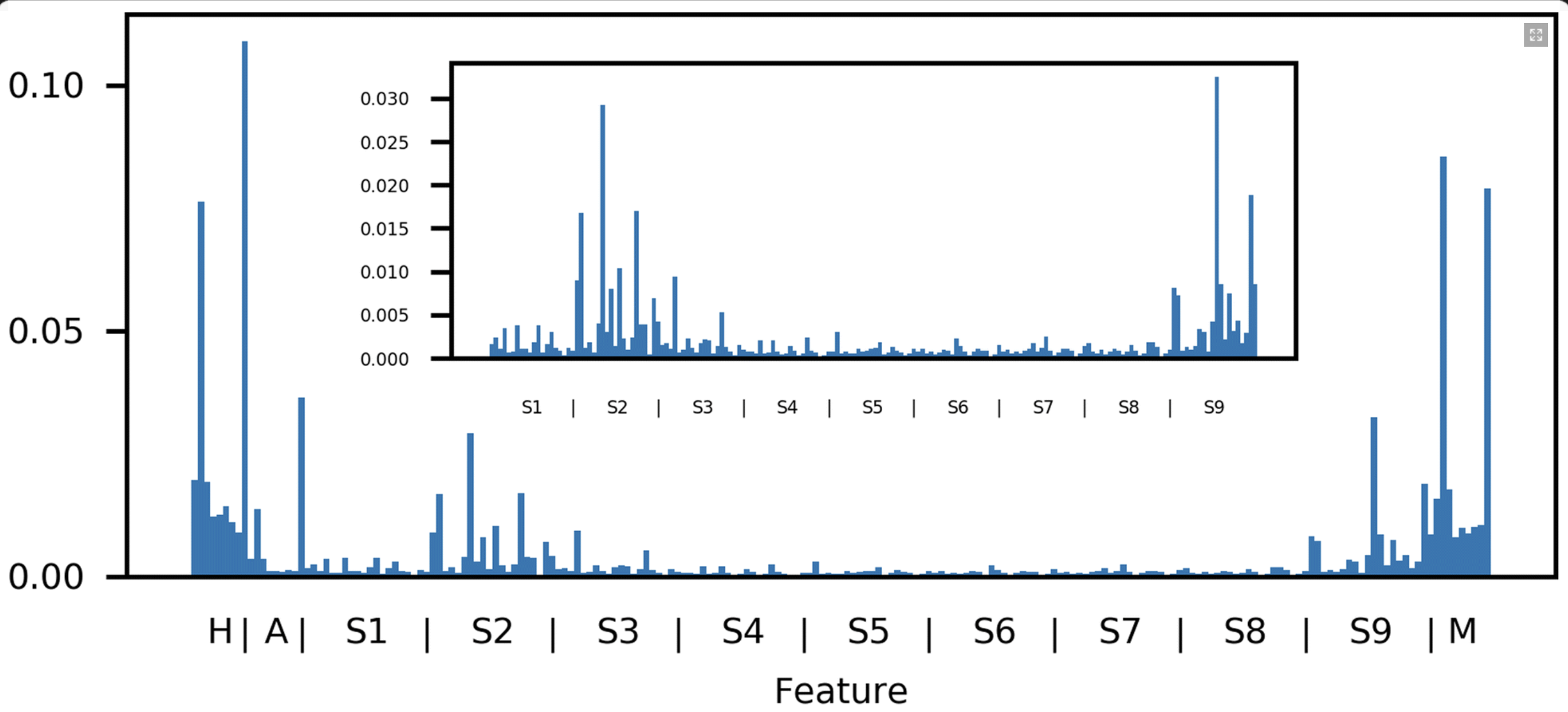
Kevin Michael Boehm and colleagues from the Englander Institute for Precision Medicine at Weill Cornell Medicine have just published a new paper on how machine learning can be used to expand our understanding of the immunopeptidome.
The new paper, “Predicting peptide presentation by major histocompatability complex class I: an improved machine learning approach to the immunopeptide,” was published on January 5, 2019 in BMC Bioinformatics.
Mr. Boehm, who is affiliated with the Weill Cornell/Rockefeller/Sloan Kettering Tri-Institutional MD-PhD Program, argued in the paper’s abstract that “improved tools are needed to identify peptides presented by major histocompatibility complex class I (MHC-I). Many existing tools are limited by their reliance upon chemical affinity data, which is less biologically relevant than sampling by mass spectrometry, and other tools are limited by incomplete exploration of machine learning approaches. Herein, we assemble publicly available data describing human peptides discovered by sampling the MHC-I immunopeptidome with mass spectrometry and use this database to train random forest classifiers (ForestMHC) to predict presentation by MHC-I.”
The authors further validated the new technique by showing that peptides predicted to bind strongly by ForestMHC correlated with stronger experimentally measured chemical affinities. This new approach to prediction outperforms established methods on newly generated data from an ovarian carcinoma cell line.
According to the authors, this new approach to testing may be used to research therapies with the potential to benefit patients since “ForestMHC has potential applicability to basic immunology, rational vaccine design and neoantigen binding prediction for cancer immunotherapy.”
# # #